Human intellect and its tenacity for invention has led to technologies that have continuously transformed the way we live and work. Why should artificial intelligence (AI) be any different, asks Associate Professor Daswin De Silva at La Trobe University’s Centre for Data Analytics and Cognition.
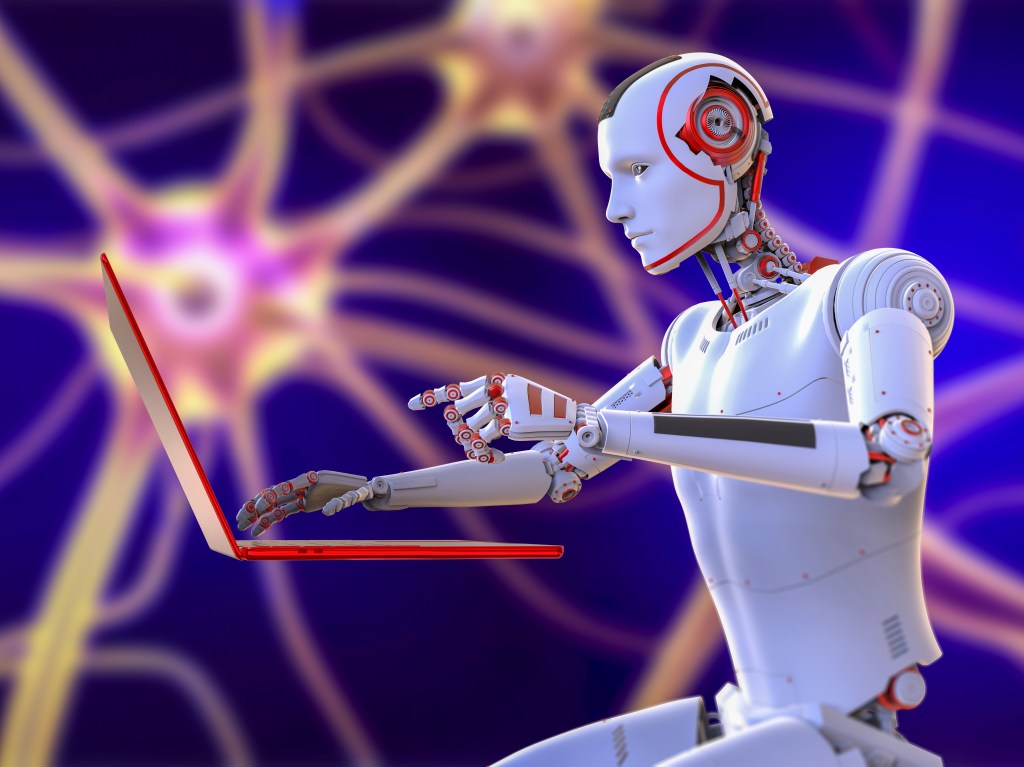
How we can learn to work with AI rather than avoiding it, asks Associate Professor Daswin De Silva at La Trobe University’s Centre for Data Analytics and Cognition.
The simple machines of wheels, levers, pulleys, industrial machines of gears, motors, engines, and digital machines of circuits, computers, clouds, are milestones of this transformation of physical and mental human labour into partial and full automation.
Remarkably, the rise of AI is a paradigm shift in this history of invention, where the automation is becoming intelligent.
Despite several failed attempts over the last sixty years, AI is substantiating its original definition coined by Professor Marvin Minsky in 1961 of ‘making machines do things that would require intelligence if done by humans.’
The most recent AI invention that is not just transforming but also disrupting most forms of knowledge work is ChatGPT by OpenAI.
Closer to home, ChatGPT is being leveraged (or exploited) by high-school and university students to automate their homework and assignments. At the other end, grading the assignments and providing descriptive feedback is also being automated by ChatGPT.
This conundrum of an ‘almost perfect’ intelligence disrupting both ends of a bilateral contract is a perfect example of why anyone not close to retirement must ‘learn to work with AI and not avoid it’.
Within the Education sector, we have effectively integrated the automation capabilities of the calculator and computer into teaching, similarly, we must now integrate the ‘automated intelligence’ of AI.
What does it mean to ‘integrate’?
Rather simply, a ‘model answer/solution’ composed by ChatGPT should be provided along with the assessment task, and the task now revised to present attempts at improving or refining this baseline with their own arguments and conclusions. This is akin to learned knowledge produced by an AI that is understood and augmented by the human intellect.
This convenient (24/7) access to engage with an equal or better intelligence is highly likely improve and expand the intellectual abilities of an entire cohort, in contrast to the time-poor, resource-constrained setting of a classroom.
Besides, knowing the utility, value and boundaries of AI from a young age, ensures the next generation of graduates are competent to work alongside the advanced machines and robots of future workplaces.
For the less enthusiastic among us who might seek to avoid or ignore AI, the wisdom of Sun Tzu is apt, “know your enemy (or in this case, workplace competition) and know yourself…”.
It is a matter of time until AI makes an imposing appearance at all workplaces; manual, physical, virtual and knowledge work.
Similar to an educational setting, it is necessary to prepare yourself by firstly, knowing what AI can and cannot do, and then leveraging AI to your advantage, by learning to use AI, build AI, collaborate with AI, or even commercialise AI.
But where do you begin to learn AI?
It is important not to be distracted by the hype surrounding artificial intelligence (AI). The first step is to contextualise learning for your needs.
But needs can be as diverse as the domains and disciplines looking to capitalise on AI, therefore, position papers can be useful in recognising the general utility of AI.
In a recent paper, An artificial intelligence life cycle: From conception to production, researchers at La Trobe University reduced all AI into four foundational capabilities; prediction, classification, association, and optimisation, while also keeping in mind each application will carry a unique noun-verb combination, such as ‘face recognition’, ‘churn prediction’, ‘emissions forecasting’, ‘customer segmentation’, ‘traffic optimisation’ etc.
Second, a general understanding of AI and its practical applications can be freely obtained by reading across several recent articles from reputable sources, endorsed by crowdsourced metrics, that document the success of using AI or provide statistics of use in diverse domains.
Beyond this generality, educational institutions and online providers offer numerous courses of varying depth and breadth, from algorithms to applications.
A logical third step is to evaluate several courses, based on expected learning outcomes, cost, time investment and testimonials, to determine a course that aligns with your needs. Finally, it is imperative to apply the learned knowledge in a practical setting, which could be a formal work task, a work task replicate, a personal project or a simple prototype of an existing solution.
Concurrently, the tools for building AI have also come of age and it is now a matter of configuring existing blocks of code instead of writing code from scratch. This also means the lead time, development and deployment times are far less than it used to be ten years ago, signifying the ‘democratisation’ of AI.
Guidelines on how to work with AI as a productivity tool where AI supports or enables decision-making in creative or aesthetic tasks
- AI output must be closely monitored to ensure cultural or social norms are adhered to,
- full stakeholder awareness, even a ChatGPT generated response to an email must include a footnote,
- continuous evaluation across multiple metrics.
Some forms of AI could also collaborate in team environments, from cobots in smart factories to the ‘devil’s advocate AI’ in the boardroom.
Such AI must possess collaborative decision-making skills and conversational/interactive skills that engage all members of the team, as well as the training, awareness, oversight and evaluation metrics noted above.
Stepping beyond workplaces, we must acknowledge that AI is also transforming our personal lives, evidenced by the numerous examples of what we consume and what we watch to how we socialise and how we vote.
It is human to fear the unknown but avoiding AI will be to our detriment. Make the next article you read, or video watched, the start of your journey into the exciting future of AI.
Daswin De Silva, PhD, SMIEEE is Associate Professor and Deputy Director at the Centre for Data Analytics and Cognition at La Trobe University
- Minsky, M. (1961). Steps toward artificial intelligence. Proceedings of the IRE, 49(1), 8-30.
- De Silva, D., & Alahakoon, D. (2022). An artificial intelligence life cycle: From conception to production. Patterns, 100489.